The Synergy Between Sensors and AI
The trajectory of advanced engineering into unprecedented territories is propelled by the confluence of sensors and Artificial Intelligence (AI), promoting advancements that seemed infeasible a few decades ago. A huge number of possibilities in different fields including industrial automation, consumer electronics, healthcare, automotive, and others are opened by this symbiotic combination. Here, we explore how sensors provide information to AI algorithms, serving as the AI's senses to understand and engage with the world.
How Sensors Provide Data to Fuel AI Algorithms
Data Acquisition and Pre-processing: In acquiring data from the environment, sensors (the basis of any responsive system) play a critical role. The raw data, reflecting various aspects of the outside world without filtering, goes through careful pre-processing to remove noise and irrelevant details. This prepares it for further analysis. The sensor data does not fit neatly into a spreadsheet or other structured format because it is usually unstructured in nature. To extract insights that would be difficult to detect manually, and to analyze unstructured data, AI algorithms can be utilized.
Feature Extraction and Selection: The crucial attributes are extracted and selected for analysis, once the data is refined. To convert the high-dimensional data into a reduced representation, focusing on the most informative characteristics, feature extraction is an imperative step. AI models are trained by feeding them the relevant features, allowing them to grasp intricate patterns and relationships present in the data.
Training AI Models: Fueled mainly by the carefully processed data from sensors, AI algorithms go through comprehensive training stages. AI models build complex mathematical relationships on the foundation provided by the data, empowering them to formulate predictions or decisions. The important determinants of the accuracy and reliability of the AI models are the quality and quantity of sensor data.
Real-time Interaction and Adaptation: The real-time interaction between AI models and the environment is facilitated by the sensors. AI dynamically adjusts and optimizes its models by continuously receiving sensor data, improving its accuracy and responsiveness. In applications such as industrial automation and autonomous vehicles where instantaneous decision-making can have significant implications, this real-time adaptation is most important.
Applications in Diverse Domains: In numerous domains, the combination of AI and sensor technology has yielded transformative results. For example, in healthcare, sensors gather essential physiological data, analyzed by AI algorithms to identify anomalies and anticipate potential health issues. Within industrial environments, sensors oversee the condition of equipment, with AI models processing the data to forecast maintenance requirements and enhance operational efficiency. Consider a manufacturing plant where sensors gather data on machine performance over time. This information can then be employed to train an AI algorithm, allowing it to predict potential machine failures.
The evolution of advanced engineering paradigms is epitomized by the seamless synergy between sensors and AI. Functioning as the sensory input for AI, sensors supply vital data, empowering sophisticated algorithms to acquire knowledge, adjust, and make well-informed decisions. This integration holds significant importance in driving ongoing technological advancements. It assures a future characterized by the continual convergence of these domains, fostering innovations that elevate the quality of life and redefine perceived limits.
Predictive Maintenance and System Optimization
The utilization of sensors alongside AI and Machine Learning (ML) has found profound applications in various industries, particularly in system optimization and predictive maintenance. This ensures the continuity and efficiency of operations. By collaborating technologically, these partnerships identify nuanced patterns and anomalies within data. This capability enables proactive maintenance interventions and the optimal allocation of resources.
Detecting Anomalies, Optimizing Operational Efficiency
Anomaly Detection: Standing out as a cardinal application arising from the synergy between sensors and AI is anomaly detection. Positioned carefully, sensors gather real-time data on the condition and functioning of machinery and systems. They adeptly identify deviations from established operational benchmarks. Processed through AI and ML algorithms, the compiled data distinguishes anomalies with increased precision, foreseeing potential system failures or disruptions. Enabling proactive interventions, this early identification minimizes downtime and prevents potential catastrophic failures.
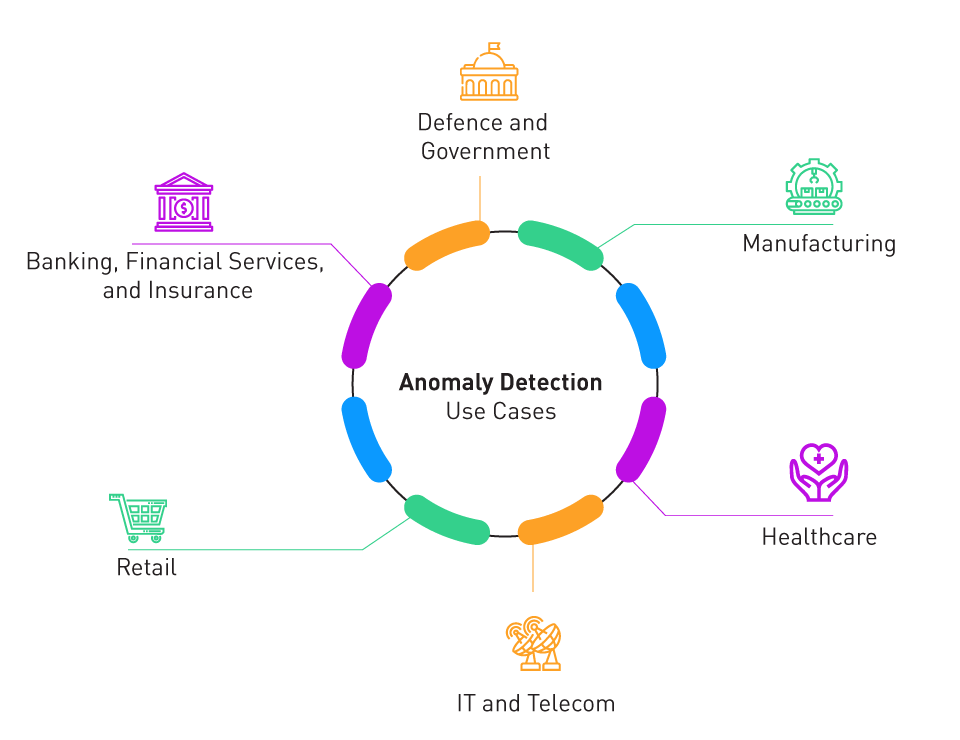
Figure 1: Anomaly detection use cases
Operational Efficiency and Resource Allocation: Apart from its role in predictive maintenance, the fusion of sensor technology with AI has implications for enhancing operational efficiency. Fueled by sensor data, AI and ML models enable a dynamic adaptation of functional specifications to current conditions, ensuring optimal performance and resource utilization. Enhancing productivity and cutting operational costs, this intelligent resource allocation and operational approach finds applications across manufacturing lines to energy grids.
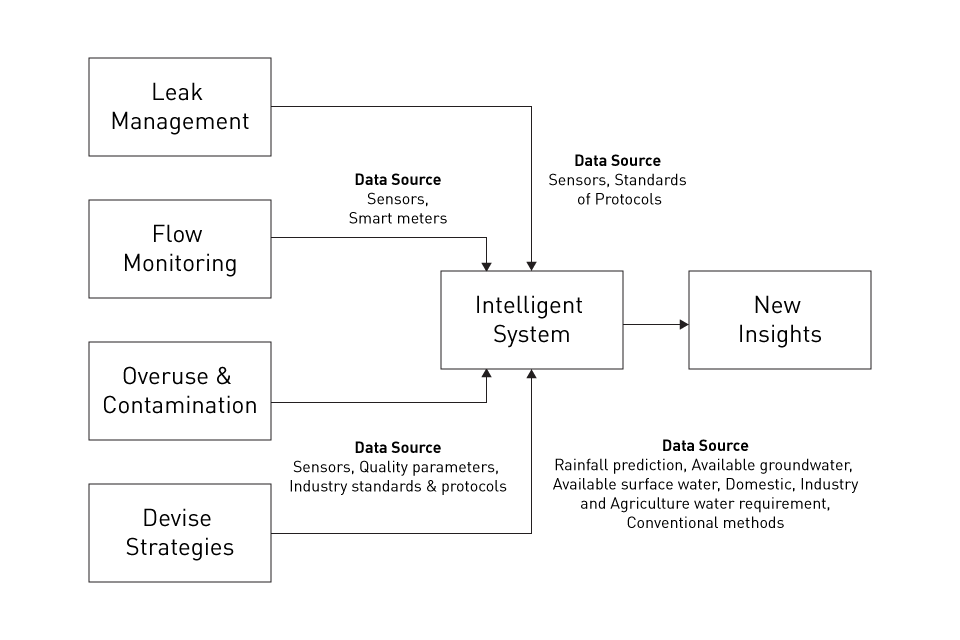
Figure 2: Harnessing intelligent systems for water management
Data-Driven Decision-Making: By integrating sensors and AI, a data-driven decision-making paradigm emerges, wherein operational strategies are guided by actionable insights derived from real-time data. Ensuring decisions are contextual, informed, and adaptive, this approach caters to the changing demands and conditions of the functional environment. Within this data-centric model, the ongoing interplay between data from sensors and analysis driven by AI refines operational processes, aiming to maximize output and minimize waste.
Applications Across Industries: Applications of predictive maintenance and optimized operations extend across various industrial landscapes. They increase production efficiency and decrease unscheduled downtime in manufacturing. They enhance optimal energy consumption and distribution along with losses mitigation in energy sectors. They also ensure the optimal and continuous function of critical life-support systems, increasing patient care and safety in the healthcare sector.
In predictive maintenance and system optimization, the symbiosis between sensors, AI, and ML has started to redefine maintenance protocols and industrial operations. Enabling early detection of anomalies and operational inefficiencies, seamless integration facilitates informed and timely interventions, along with optimal resource allocation. With the continuous evolution of technology, the heightened reliability and efficiency resulting from these innovations are poised to emerge as the cornerstone of industrial and technological progress. This will propel industries into a new era characterized by operational excellence.
Real-time Analytics and Decision Making
At the core of AI and Machine Learning applications, harmonized with sensory data, lies the essence of real-time analytics and immediate decision-making. This forms an intelligent framework capable of promptly responding to dynamic operational environments. Navigating the complexities of real-time data is crucial in various applications, ranging from autonomous vehicles to critical healthcare systems, highlighting the essential dynamism inherent in this integration.
The Importance of Fast Sensor Data Processing
Speed and Accuracy: Real-time analytics relies significantly on swift sensor data processing, guaranteeing the accuracy and timeliness of decisions and responses produced by AI and ML models, making it a pivotal component. Ensuring the insights gleaned mirror the current state of the environment, swift and precise processing of sensor-derived data facilitates instant adaptation and response to emerging conditions and requirements.
Enhanced Responsiveness: Enhanced responsiveness of systems is empowered by the ability to rapidly process sensor data and generate real-time analytics. In situations like autonomous driving or industrial automation, where circumstances can change rapidly, the capability to rapidly interpret and respond to sensor data can be the determining factor for optimal functioning and operational failure. It can even prevent potential accidents and system malfunctions.
Optimizing Decision-Making Processes: Enriching decision-making processes, fast sensor data processing empowers AI models to analyze data streams in real-time, facilitating instant and informed decision-making. Maintaining operational fluency in dynamic environments hinges on the immediacy of decision-making. This allows systems to instantly adapt their actions to accommodate evolving conditions, ultimately optimizing overall system performance and reliability.
Applications and Implications: The collaboration between real-time analytics and rapid sensor data processing has diverse applications. In healthcare, it facilitates ongoing monitoring and prompt responses to changes in patient conditions. Within industrial automation, it enables immediate adaptations to production parameters when faced with fluctuations in input variables. Meanwhile, in smart cities, this technology supports real-time traffic management and resource allocation, contributing to enhanced urban livability and sustainability.
The fundamental to unleashing the full potential of real-time analytics and decision-making is the combination of swift sensor data processing with AI and Machine Learning. Ensuring systems are aware of their environment and can respond in real time, this amalgamation optimizes operational efficiency, responsiveness, and reliability across various applications. Advancing technology will persistently reshape the interaction and response paradigms between systems and their environments, as the intersection of real-time data processing and intelligent analytics continues to herald new frontiers in technological innovation and application.
Case study: AI-Driven Health Monitoring System Using Bio-Sensors
Revolutionizing healthcare, the emergence of AI-powered health monitoring systems equipped with bio-sensors introduces a paradigm shift. These systems provide unparalleled capabilities in patient monitoring, diagnostics, and predictive analysis. At the intersection of AI and sensory technologies, these systems embody the fusion that is reshaping healthcare experiences, outcomes, and accessibility, offering transformative solutions.
The System Architecture: Centered on bio-sensors, an AI-driven health monitoring system is fundamentally crafted to capture a diverse range of physiological parameters, including body temperature, heart rate, blood pressure, and more. Subsequently, the collected data is inputted into sophisticated AI models with the capability to interpret intricate physiological data in real-time. This empowers prompt responses and interventions upon the detection of anomalies.
Real-Time Health Monitoring: Providing continuous and real-time health monitoring, bir-sensors are crucial to the AI-driven system. Capturing crucial health parameters, these systems transmit the data to integrated AI algorithms for processing. In real-time, any deviations from the norm are promptly identified, facilitating immediate diagnostics and intervention as necessary. This proactive approach helps prevent potential health crises.
Predictive Analysis and Preventative Action: Going beyond instant response, the collaboration between bio-sensors and AI enables predictive analysis, detecting potential health risks before they manifest as medical conditions. Facilitating a preventive strategy, healthcare providers can recommend timely interventions and lifestyle adjustments, potentially thwarting the development of chronic conditions and enhancing both overall patient health and quality of life.
Personalized Healthcare Solutions: Personalized healthcare solutions designed to individual health profiles and needs are offered by an AI-driven health monitoring system using bio-sensors. By learning and adapting to the distinct physiological parameters of each user, the system guarantees enhanced precision and effectiveness in health monitoring and management. This cultivates an atmosphere conducive to personalized healthcare and wellness strategies.
Scalability and Accessibility: Through the smooth integration of bio-sensors and AI, not only is health monitoring optimized, but healthcare also becomes more scalable and accessible. AI's automation and precision not only alleviate the burden on healthcare providers but also broaden access to quality healthcare by making health monitoring available to larger populations, thereby democratizing quality healthcare access.
Illustrating the transformative potential, this case study showcases the integration of AI and bio-sensors in healthcare. Elevating the precision and effectiveness of health monitoring, an AI-driven system propels healthcare into a new era characterized by predictive analysis and personalized medicine. Leveraging the synergies between AI and bio-sensors, these systems are forging a path towards healthcare solutions that are proactive, preventative, and centered around the patient. They redefine the paradigms of health and wellness, setting new standards for future generations.
直接登录
创建新帐号