Upcoming Innovations in Power Conversion Technology
Power conversion technology is poised for considerable breakthroughs, driven by the growing demand for efficient, reliable, and sustainable energy solutions. As the world moves toward cleaner energy sources and smarter grids, advances in power conversion technologies will become increasingly vital. This section delves into some of the most promising upcoming advancements in power conversion technology, focusing on wide bandgap semiconductors, innovative topologies, energy efficiency gains, and the integration of power conversion with growing digital technologies.
Wide Bandgap Semiconductors
Silicon carbide (SiC) and gallium nitride (GaN) are two examples of wide bandgap (WBG) semiconductors, which are among the most significant innovations in power conversion technology. When compared to traditional silicon (Si) semiconductors, these materials have better electrical properties that allow for higher efficiency, higher power density, and improved thermal performance.
Key Advantages:
- Higher Efficiency: Reduced conduction and switching losses result from WBG semiconductors' higher switching rates and lower on-state resistance. As a result, power converters are more efficient overall.
- Higher Operating Temperatures: Compared to silicon-based devices, WBG devices can operate at significantly higher temperatures, which eliminates the need for massive cooling systems and allows for more reliable and compact designs.
- Increased Power Density: More compact power converter designs are made possible by the ability to function at higher voltages and frequencies. This is especially advantageous in applications with limited space, like electric vehicles and aerospace systems.
Applications: WBG semiconductors are being used more and more in a range of applications, including as high-efficiency power supplies for consumer and industrial electronics, renewable energy inverters, and electric vehicle (EV) powertrains. As the cost of these materials declines, their use is anticipated to accelerate, resulting in additional advancements in power conversion.
Advanced Converter Topologies
More advanced topologies that provide better performance in terms of efficiency, power quality, and scalability are complementing and, in some cases, replacing traditional power converter topologies like the two-level inverter. Advanced topologies that are becoming more popular include matrix converters, resonant converters, and multilevel converters.
Multilevel Converters: Multilevel converters enhance power quality and lessen harmonic distortion by generating output waveforms with multiple voltage levels. High-voltage, high-power applications like HVDC transmission and extensive integration of renewable energy sources are especially well-suited for them. Multilevel converters are becoming more economical and efficient due to advancements in semiconductor devices and innovative control strategies.
Resonant Converters: In order to provide soft switching and lower switching losses and electromagnetic interference (EMI), resonant converters utilize the inherent resonance of inductive and capacitive components. Applications like wireless power transfer and portable electronics that demand great efficiency and low noise levels are perfect for these converters.
Matrix Converters: Compact designs and increased efficiency are the results of matrix converters' direct AC-to-AC conversion, which eliminates the need for intermediate DC stages. Recent developments in digital control and semiconductor technology are opening up a larger range of applications for matrix converters, despite their historical limitations due to complex control requirements.
Energy Efficiency Improvements
Improving energy efficiency is a primary driver of innovation in power conversion technologies. There is a growing need to minimize losses at all stages of power conversion, from generation to end-use, as energy consumption continues to rise.
Bidirectional Converters: A significant trend in energy efficiency is the development of bidirectional converters. Applications such as energy storage integration with the grid and regenerative braking in electric vehicles are made possible by these converters, which enable the smooth flow of energy in both directions. Bidirectional converters lower losses and increase system efficiency by streamlining the energy flow.
Digital Control and Optimization: Real-time optimization of power conversion systems is being rendered by the integration of digital control techniques, such as Model Predictive Control (MPC) and algorithms based on Artificial Intelligence (AI). These advanced control techniques can adjust to changing conditions, minimize losses, and guarantee that the system operates at maximum efficiency.
Power Factor Correction (PFC): PFC technology innovations focus on reducing reactive power and harmonics to increase power supply efficiency. Active PFC circuits are increasingly found in power supplies for everything from industrial machinery to consumer electronics. These circuits dynamically modify the input current to match the voltage waveform.
Integration with Emerging Digital Technologies
New potential for innovation are being created by the combination of power conversion technology with cutting-edge digital technologies such as advanced sensors, artificial intelligence (AI), and the Internet of Things (IoT). Smarter, more responsive, and more efficient power conversion systems are made possible by these technologies.
Smart Grids: Power converters with digital control and communication capabilities can communicate with the grid in real-time in smart grid applications, modifying their operation in response to demand patterns, grid conditions, and renewable energy availability. This dynamic interplay minimizes losses, optimizes energy distribution, and improves grid stability.
AI and Machine Learning: Power conversion systems are utilizing AI and machine learning to facilitate real-time optimization, fault detection, and predictive maintenance. AI algorithms can identify patterns and predict potential failures before they happen by evaluating enormous volumes of operational data, which lowers downtime and increases system reliability.
IoT Integration: The integration of power converters with IoT devices enables improved monitoring, control, and data collection. Through communication with other devices in a network, IoT-enabled power converters allow for more accurate control over load balancing, energy consumption, and grid interaction. This is especially important in smart homes and distributed energy systems, where efficient management of energy resources is required.
Ultra-High Frequency Power Conversion
Another area of innovation is the drive for ultra-high frequency (UHF) power conversion. UHF converters, which operate at frequencies higher than 1 MHz, have the potential to be significantly lighter in weight and smaller in size, while also being more efficient.
Benefits:
- Smaller Passive Components: The converter's overall size and weight can be decreased by using smaller inductors and capacitors at higher operating frequencies.
- Faster Response Times: UHF converters improve dynamic performance by responding faster to changes in input conditions or load.
- Lower Losses: UHF converters can improve efficiency by lowering the amount of time switches spend in transition states.
Challenges: Improvements in semiconductor technology, temperature control, and electromagnetic compatibility (EMC) are necessary for the development of UHF converters. However, continued research and development is making these converters more and more feasible for applications like military, aerospace, and portable electronics where efficiency, weight, and compactness are crucial considerations.
The Impact of Artificial Intelligence and Machine Learning on Control Systems
Artificial intelligence (AI) and machine learning (ML) are transforming the field of control systems, particularly in power electronics and energy management. Traditional control strategies are often inadequate to manage the dynamic, non-linear, and high-dimensional nature of power systems, which is becoming increasingly complicated as renewable energy sources, electric vehicles, and smart grid technologies are being integrated. By enabling more intelligent, adaptable, and efficient control systems, AI and ML provide strong tools to address these challenges. This section explores how AI and ML impact control systems, emphasizing important applications, benefits, and potential future developments.
Enhanced Predictive Control
Predictive control is one of the areas where AI and ML have made the biggest contributions to control systems. In order predict future system behavior and optimize control operations, traditional Model Predictive Control (MPC) uses mathematical models. However, the accuracy and ability of these models to deal with complex, non-linear systems can be limited. By offering more precise and flexible models based on data-driven methodologies, AI and ML can improve predictive control.
Machine Learning in Predictive Control: ML techniques, particularly those based on neural networks, can be trained on massive datasets to simulate complex system dynamics that are difficult to represent using traditional methods. These models can be used to optimize control actions and predict system behavior in real-time once they have been trained. An ML-based predictive controller, for instance, can predict how weather-related fluctuations in power output will affect a renewable energy system and modify the operation of inverters, energy storage, and other components accordingly.
Benefits: Predictive control using AI and ML increases prediction accuracy, strengthens the ability to handle non-linearities, and enables more complex control action optimization. As a result, the system performs better, uses less energy, and is more flexible in response to changing conditions.
Adaptive and Self-Learning Control Systems
Traditional control systems frequently rely on predefined rules to regulate system behavior and are designed with fixed parameters. This approach might not be enough in systems that are prone to rapid fluctuations and uncertainties, however, it can be effective in environments that are steady and predictable. Adaptive and self-learning control systems that can automatically modify their parameters and strategies in response to real-time data are made possible by AI and ML.
Reinforcement Learning: Reinforcement learning (RL) is a key machine learning technique used in adaptive control. Through interaction with the system and feedback in the form of rewards or penalties, RL algorithms learn optimal control strategies. The RL agent gradually improves system performance by modifying its actions to optimize rewards. RL can be applied to power electronics to enhance the performance of converters, inverters, and other components in response to changing grid stability needs, energy prices, and load conditions.
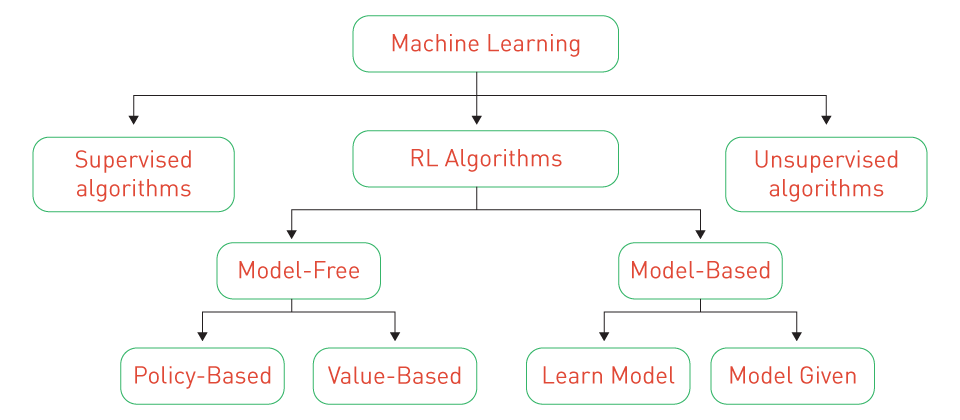
Figure 25: ML algorithms
Benefits: Adaptive and self-learning control systems provide various advantages, including higher resilience to disturbances, increased efficiency in dynamic environments, and a reduced need for human intervention. These systems can constantly enhance their efficiency over time, making them ideal for use in smart grids, renewable energy systems, and self-driving automobiles.
Fault Detection and Predictive Maintenance
The way control systems handle fault detection and maintenance is changing as a result of AI and ML. Conventional fault detection techniques often rely on threshold-based techniques, which can generate false alarms or miss subtle signs of new issues. By examining vast volumes of sensor data to find patterns and abnormalities that indicate possible failures, AI and ML enable sophisticated fault detection.
Predictive Maintenance: Predictive maintenance reduces downtime and enables proactive maintenance by using AI and ML to predict equipment failures before they happen. Machine learning algorithms can forecast when a component is likely to fail and suggest maintenance procedures to avoid unplanned malfunctions by evaluating historical data and real-time sensor inputs. This can involve monitoring the condition of capacitors, semiconductors, and other crucial components in power electronics.
Benefits: Integrating AI and ML into fault detection and predictive maintenance systems improves reliability, lowers maintenance costs, and increases equipment life expectancy. By only scheduling maintenance when necessary, rather than on a fixed schedule, it also makes it possible to use resources more efficiently.
Optimization of Energy Management Systems
In a power system, energy management systems (EMS) are in charge of maximizing energy production, distribution, and consumption. Traditional EMS approaches struggle to achieve maximum performance as energy systems become more complicated due to the integration of energy storage, electric vehicles, and distributed energy resources (DERs). AI and ML present new opportunities to improve EMS's optimization capabilities.
Energy Forecasting: AI and machine learning algorithms are extremely effective at predicting energy demand, renewable energy generation, and power costs. When it comes to storing energy, selling it back to the grid, and balancing supply and demand in real time, EMS can make better decisions with precise predictions. AI-based forecasting, for instance, can assist in balancing end-user consumption patterns with the fluctuating output of solar and wind energy in a smart grid.
Real-Time Optimization: Real-time optimization of energy flows in complex systems is another application for AI and ML. Through continuous analysis of data from sensors, meters, and other sources, AI algorithms can modify the operation of energy storage systems, generators, and inverters to maximize the use of renewable energy, minimize losses, and lower costs. This is especially crucial in distributed energy systems and microgrids, where dynamic optimization is essential.
Benefits: EMS that uses AI and ML increases energy systems' flexibility and efficiency, facilitates the integration of renewable energy, and assists in the transition to a more sustainable energy future. These technologies offer more intelligent and responsive energy management, which is essential for smart grid operation and successful DER integration.
Integration with IoT and Cyber-Physical Systems
The confluence of AI, machine learning (ML), and the Internet of Things (IoT) is enabling the development of complex cyber-physical systems (CPS) that combine physical processes with digital control and communication. This integration allows for more precise monitoring, control, and optimization of energy flows across distributed systems in power electronics and energy systems.
Smart Sensors and Actuators: IoT devices that have intelligent sensors and actuators installed are able to gather comprehensive data on power system performance and transmit it to AI-based control systems for analysis. In order to maximize system performance, the AI algorithms analyze the data, find patterns, predict future conditions, and make modifications in real time.
Cybersecurity Considerations: Maintaining the cybersecurity of control systems is crucial as AI and ML are incorporated into these systems more and more. Strong security features must be incorporated into AI-based controls to guard against online attacks that can jeopardize power systems' operation.
Benefits: Power systems can operate more efficiently, reliably, and securely because of the integration of AI, ML, and IoT in CPS. It also encourages the development of autonomous systems that can function with minimal assistance from humans, adjust to changing conditions, and respond instantly to new threats in real-time.
直接登录
创建新帐号